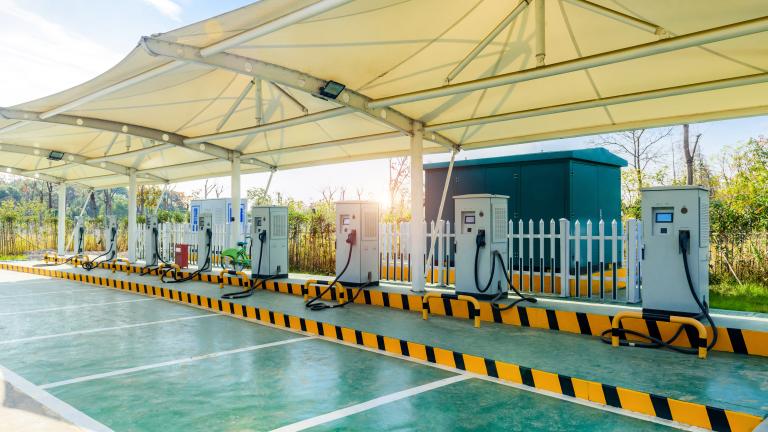
Significant public resources are being dedicated to stimulating private sector investment in electric vehicle (EV) charging infrastructure. In the U.S., firms can access grants made available by the recently passed Infrastructure Investment and Jobs Act and Inflation Reduction Act. The question this research addressed is how state and local governments can make the most of such public funding to accelerate investment in fast charging stations for EVs.
A key goal for governments is incentivizing fast charging stations in currently underserved rural areas. Such investments present challenging economics for private sector investors due to high upfront costs in combination with low and uncertain demand. For firms that can choose when to invest, investment carries an opportunity cost, which incentivizes delaying development until demand is sufficiently high.
To effectively accelerate investment, subsidy design should account for the full set of incentives facing investors. For this purpose, we develop a real options model of investing in a representative fast charging station in the U.S. Our model captures optionality in investment timing, thus allowing us to quantify optimal investment timing, which previous analyses omitted due to a reliance on simpler Net Present Value (NPV) methods.
We model the investment in a single fast charging station comprising six 350 kW charging points. Demand for charging is assumed to be low in the first year, and for this we use a typical low utilization rate of 5%. Future demand growth is uncertain, which we represent as a binomial scenario tree with an average annual demand growth of 9% and volatility based on historical charging data. We assume the investor considers a decision-making horizon between now and ten years in the future and must choose the point in time within this horizon at which to invest. Our model computes the optimal investment decisions for each possible demand level and at each possible point in time using a standard backward recursion algorithm. We then estimate the optimal timing of investment by simulating many possible future scenarios using a Monte Carlo algorithm.
Our analysis shows that optionality in investment timing has substantial impact on the effectiveness of government subsidies and important implications for subsidy design. We first demonstrate that subsidies informed by traditional NPV methods either underestimate the amount of subsidy required to trigger investment or overestimate the effectiveness of the subsidy. An NPV analysis would recommend a subsidy large enough for the project to break even so that its discounted future revenues equal its costs. For the case we consider, this would result in a grant equal to 86% of the investment cost (shown by the red dot in the figure below). This large magnitude is largely driven by the combination of high investment costs, low demand, and substantial demand charge costs (as well as our choice to omit any revenue sources other than the re-sale of electricity). However, such a grant would not cover the opportunity cost of investment (shown by the blue line). For the project to justify this additional cost, our model estimates that a grant equal to 160% of the project’s investment cost becomes necessary (shown by the blue dot in the figure), or roughly twice as much as the subsidy recommended by NPV. On the other hand, if the investor only received the NPV-informed grant, our model suggests that it would be optimal for the investor to wait more than 5 years before investing.
Figure 1. Value of investment in a fast charging station relative to the size of a grant subsidy
This research suggests several policy design changes that can improve the effectiveness of charging subsidies in the presence of optionality. A recently proposed option is for governments to provide long-term contracts that provide investors with guaranteed revenue streams, a version of which has been implemented in the Netherlands. A specific policy we test is a two-sided contract for differences, which compensates firms for any revenue shortfalls below a pre-determined “strike” level and requires firms to pay back any revenues in excess of this level. We find that, by directly addressing optionality, such contracts can effectively accelerate investment. We further estimate that such contracts are substantially more cost-effective than grants, requiring less financial support to trigger investment.
A simple policy alternative is the introduction of a phase-out schedule for subsidy grants. This would provide a considerable improvement in cost-effectiveness (compared to the standard grant) by decreasing the value of delaying investment. We test alternative phase-out timelines and find that a 10-year phase-out schedule may be a pragmatic way to cost-effectively accelerate charging investments.
Surprisingly, this paper shows that reducing (but not eliminating) investment risk has relatively little impact on investment timing. To explore this, we test a policy such as a Zero Emission Vehicle standard that mitigates EV adoption risk by effectively mandating a given level of EV penetration. In this case, firms continue to face some risk (e.g. related to driving behavior), and our real options model suggests that the optimal investment decision is changed only slightly. This suggests that effective de-risking would require that governments address residual risks additional to the uncertainty in EV adoption. In practice, ZEV mandates can still play an important role in charging infrastructure policy. Our analysis only represented ZEV standards as a reduction in the uncertainty in future EV adoption. But if such policies increase EV adoption, they would by extension have a positive effect on charging investments.
The relevance of our analysis is limited to cases where firms have the option to delay investing. This is particularly likely to be the case in low-demand rural regions. Therefore, analyses informed by real options can help public agencies understand and stimulate investment decisions in areas that may otherwise be underserved, reducing inequalities in vehicle electrification and more effectively alleviating range anxiety concerns.
Further Reading:
CEEPR WP 2023-03
About The Authors
![]() |
Emil Dimanchev is a Research Affiliate at MIT CEEPR and a Ph.D. Candidate in Electric Power Engineering at the Norwegian University of Science and Technology. He has testified before legislators and advised government officials on climate policy in the U.S. and the European Union. He was previously a Senior Carbon Market Analyst at Thomson Reuters Point Carbon, where he advised Members of the European Parliament and member state officials on the reform of the European Union Emissions Trading System. He holds a Master’s degree from the MIT Technology and Policy Program (TPP ’18). |
![]() |
Stein-Erik Fleten received the Ph.D. degree in operations research from the Norwegian University of Science and Technology, Trondheim, Norway, in 2000. He is currently a Professor at the Norwegian University of Science and Technology, Trondheim, Norway. His areas of interest include electricity markets, hydro scheduling under uncertainty, hedging and risk management in electricity utilities, real options, and bidding in short-term electricity auctions. His main scientific fields are stochastic programming, finance and energy economics, with applications for electricity companies. He has been doing research on the liberalized electricity market since the 1990s. He has been a visiting scholar at the University of British Columbia, University College London and the University of Michigan. Fleten has extensive experience in participation and leadership of applied research projects, both nationally and internationally, within the electricity area. |
![]() |
Don MacKenzie is an Associate Professor of Civil & Environmental Engineering and leads the Sustainable Transportation Lab at the University of Washington. He earned a Ph.D. in Engineering Systems from the Massachusetts Institute of Technology, and his research focuses on how new technologies, user behavior, and policy can be directed to make our transportation system more economically viable and environmentally benign, while providing access to opportunities for all. He is the Editor of Energy Findings, a member of the Transportation Research Board’s Standing Committee on Transportation Energy, and serves on the editorial board of Transportation Research Part D: Transport and Environment. |
![]() |
Magnus Korpås is a Professor in Electric Power Engineering at the Norwegian University of Science and Technology (NTNU), where he established the research group on Electricity Markets and Energy System Planning. His research interests include renewable energy, energy storage, power markets and energy system integration. He received his Ph.D. degree from NTNU in 2004 and he is a former Research Director at SINTEF Energy Research. Currently he is main supervisor for 10 Ph.D. students, teaches a graduate course in power markets and participates in several collaborative energy research projects at national and European level. In 2018-2019, he was a visiting scholar at the MIT Laboratory of Information and Decision Systems (LIDS). |